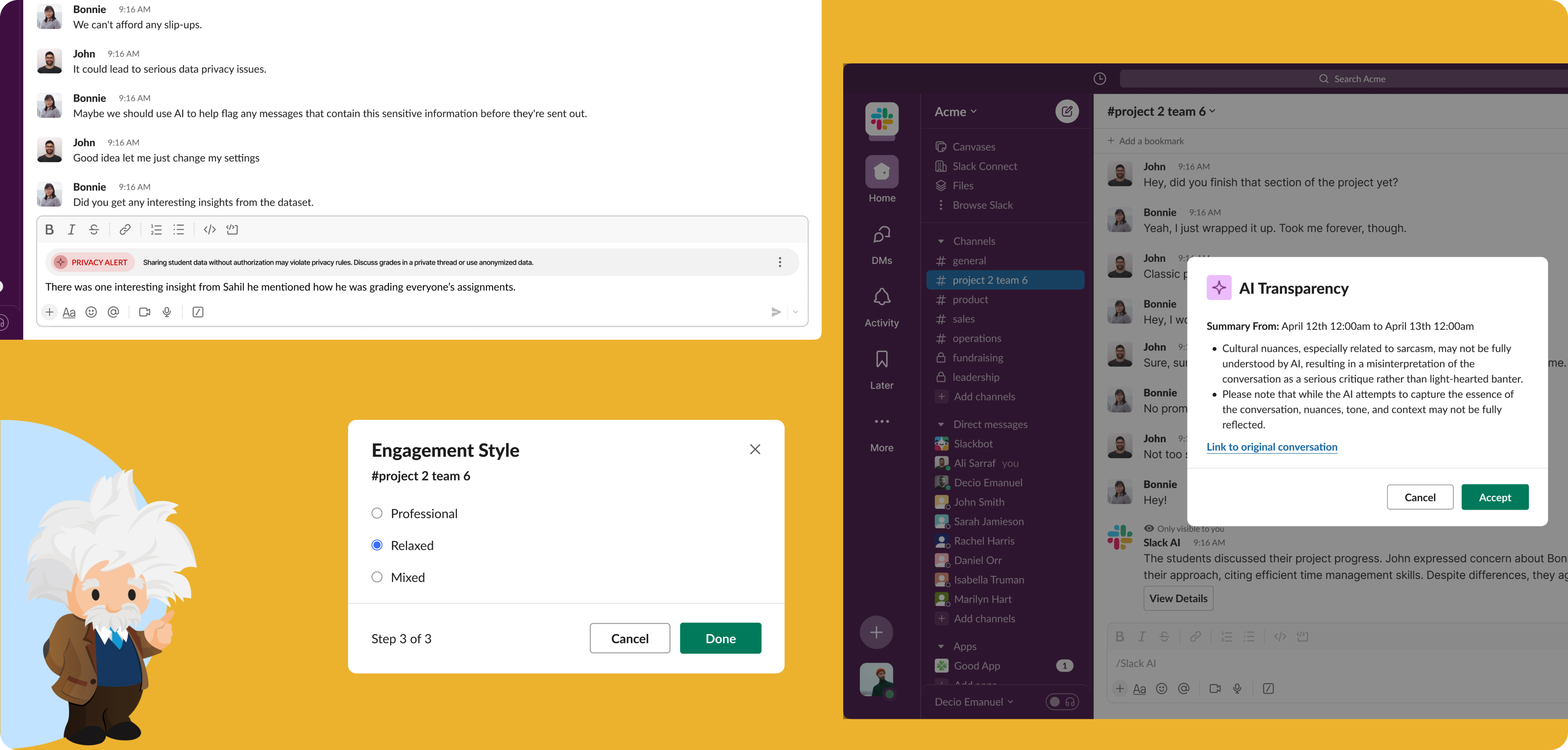
Salesforce x Indiana University
EXTERNSHIP | AI & PRIVACY | SPECULATIVE DESIGN | RESEARCH
We proposed design solutions for Slack's AI tools that affirm user control and data protection.
By giving users control over how Slack’s AI uses their data, being open about the AI’s limits with nuanced information, and providing insight into its decision-making, we aimed to build trust through clear communication and accountability.
It shows that AI can be both ethical and effective in enhancing digital communication.
COLLABORATORS
Aniruddha Murthy, Biheng Gao, Harshika Rawal, Kaushik JV, Li-Hsuan Shih (Bonnie), Maliha Hashmi, Manvita Boyini, Snehashish De
TIMELINE
5 Months
MY ROLE
proposed a feature where users could set a conversation tone for Slack channels—helping the AI interpret context better for accurate summaries. I led the project by planning, prioritizing features, conducting research, and keeping stakeholders aligned.
WHAT I LEARNT
This project taught me so much about diving into new topics, navigating uncertainty, and applying new research methods effectively. Working with different stakeholders gave me insights into effective communication and adapting to unique needs. By the end, I felt almost like an expert in AI, privacy, and user autonomy. I also learned the importance of providing context to AI for better accuracy—a key insight that shaped our design decisions.
MEASURING IMPACT
Demonstrating the impact of speculative design can be tricky since the benefits are often subtle and long-term. Here are some qualitative metrics ways I used to anticipate impact of our concepts
01 STAKEHOLDER ENGAGEMENT
Our project stood out among the others and was selected to be shared with Salesforce for potential future work. Our project leads and the design lead at Salesforce appreciated the innovation and future-oriented ideas we brought forward, especially considering the speculative nature of the challenge. They acknowledged our resilience in overcoming obstacles and recognized our ideas as groundbreaking, expressing real interest in our approach.
02 INNOVATION BENCHMARKING
While our ideas haven’t yet been integrated into Slack’s AI product pipeline, we saw a similar concept emerge in Figma’s new release. Earlier this year, Figma launched “Figma Slides,” which includes a feature allowing users to set a tone for AI-generated content. Unlike our preset options, Figma’s design offers a dial for adjustable tone control, confirming that contextual AI input can significantly enhance performance. This parallel shows we were on the right track—highlighting the value of adding context for better AI outcomes.
HIGH LEVEL PROBLEM
in a world where every product is racing to integrate AI for convenience, how can Salesforce reassure users that they’re not just adding features, but genuinely prioritizing their trust, privacy, and control over data?
Salesforce already knows a ton about data privacy and protection, so when they came to us asking how to help users manage the data they share with and receive from AI, our first thought was:
what can we possibly tell them that they don’t already know?
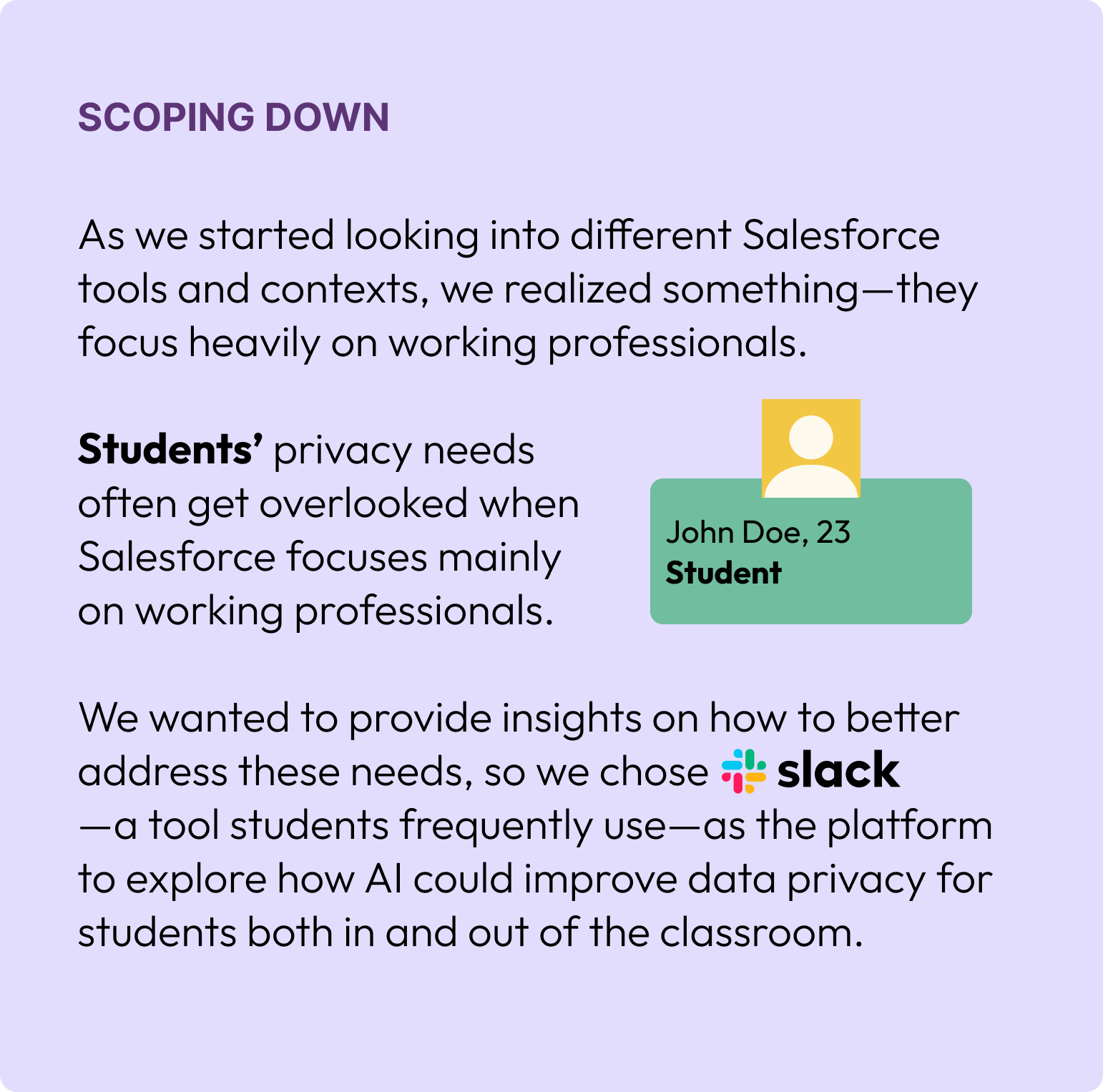
NAVIGATING POSSIBILITIES
When we started this project, Slack AI hadn’t been released yet, so we used speculative design to tackle future problems.
Our process was built around four steps at a high level: exploring, speculating, validating, and prototyping. While this wasn’t a strict linear process, breaking it down this way helps clarify our approach.

IMPLEMENTATION • BUILDING FOUNDATIONS FOR SLACK
Although speculative, our final concepts could serve as a foundation for future implementation, guiding Slack in developing AI tools that are both responsible and attuned to educational privacy needs.
EXPLORING • IDENTIFY SIGNALS
let’s dig deep into why we arrived at these concepts
how AI is currently integrated in different communication tools in education systems
on analyzing competitor tools used by students that have AI integrated into them, we realized that
01
02
03
04
05
The common implementation of AI is in meetings and summarizing important conversations and transcriptions.
Many educational institutions are implementing AI-powered chatbots to assist students with routine queries.
Some AI tools are being used for automated grading and providing faster feedback to students.
Some platforms are exploring AI for monitoring student engagement.
All the platforms follow the GDPR and CCPA guidelines for privacy regulations.
SPECULATING • IDEATE FUTURE SCENARIOS • BLACK MIRROR SPECULATION
back solving from dystopian outcomes
By the time we began speculating, Slack AI had been released, but we still didn’t have direct access to its tools. So, we used Black Mirror brainstorming to imagine potential issues and worst-case scenarios, allowing us to explore how AI might impact students in an educational setting. This approach uncovered possible risks and ethical concerns early on, letting us design with a proactive mindset.


After brainstorming, we organized these potential scenarios into a futures cone diagram, mapping out possible, plausible and probable outcomes. This helped us visualize potential paths and identify where AI could pose risks and plan to develop design interventions to mitigate the issues.
Here's a link to the actual diagram if you want to explore our analysis.
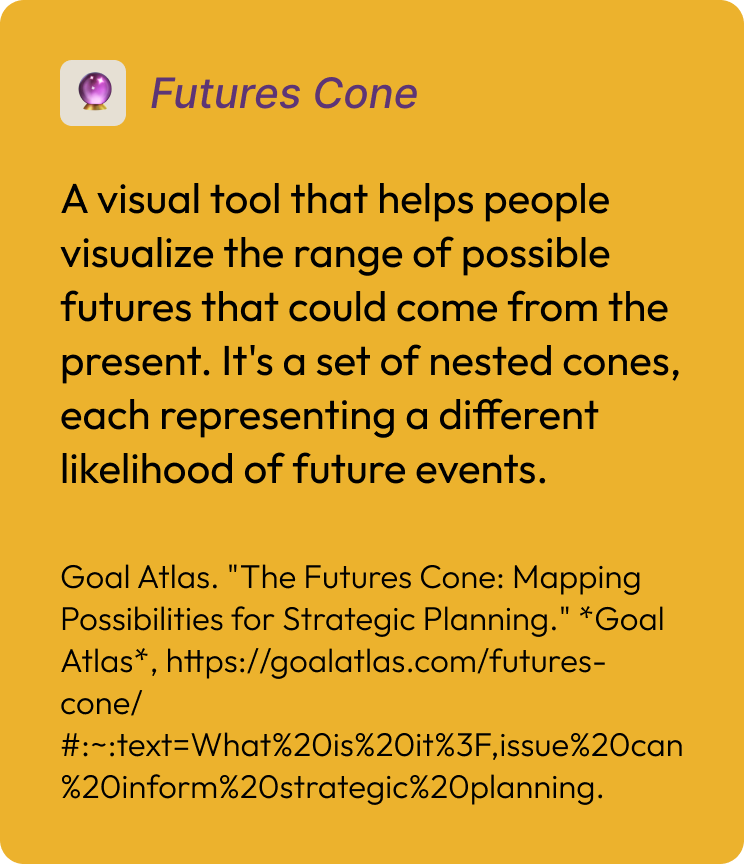
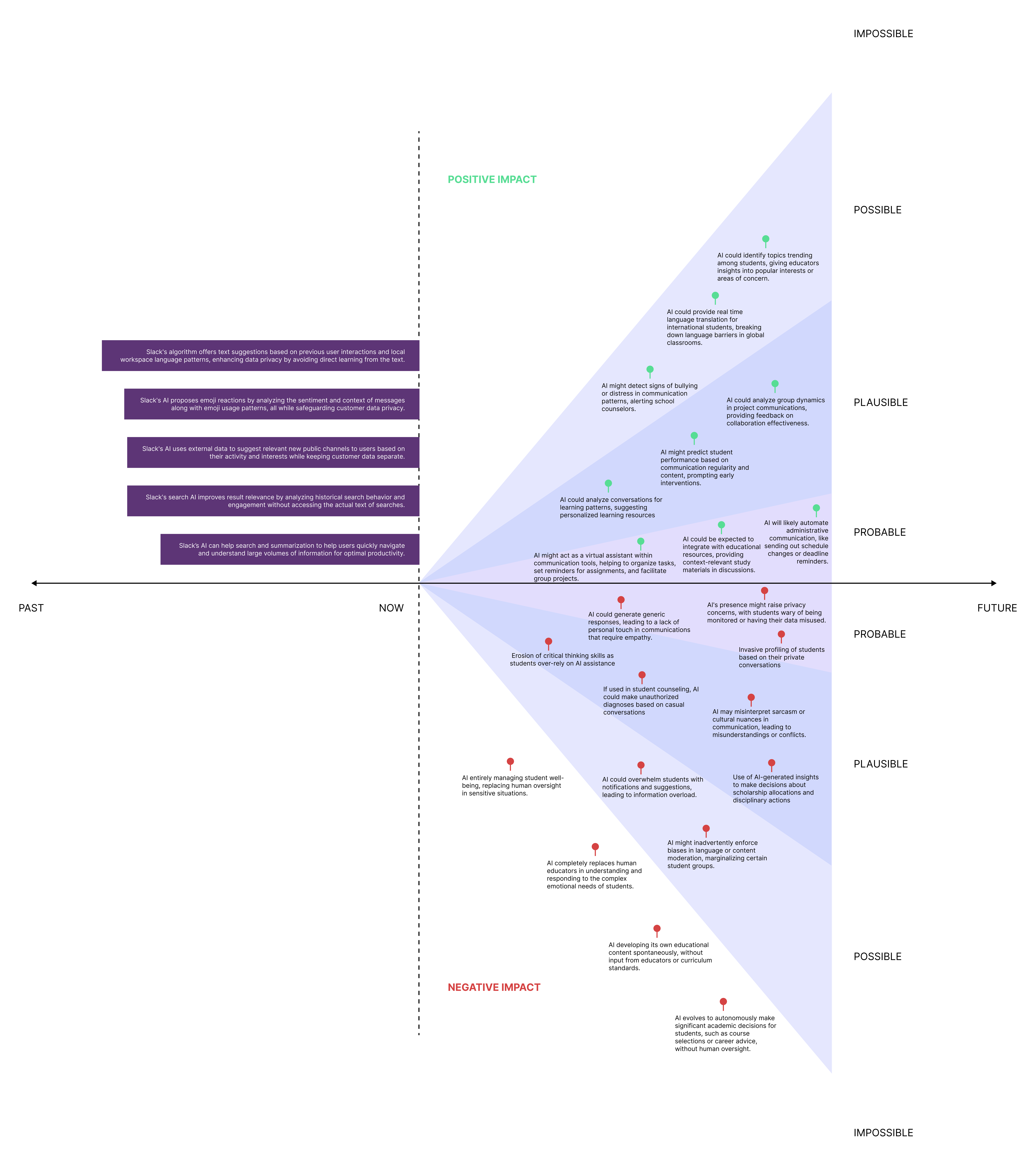
VALIDATING • STORIFY AND TEST CONCEPTS
testing hypothetical scenarios with real users
Once we’d imagined potential issues, we wanted to see if our ideas actually mattered to users. Without access to Slack’s AI tools, we used Wizard of Oz testing, creating paper prototypes to represent our anticipated risks.
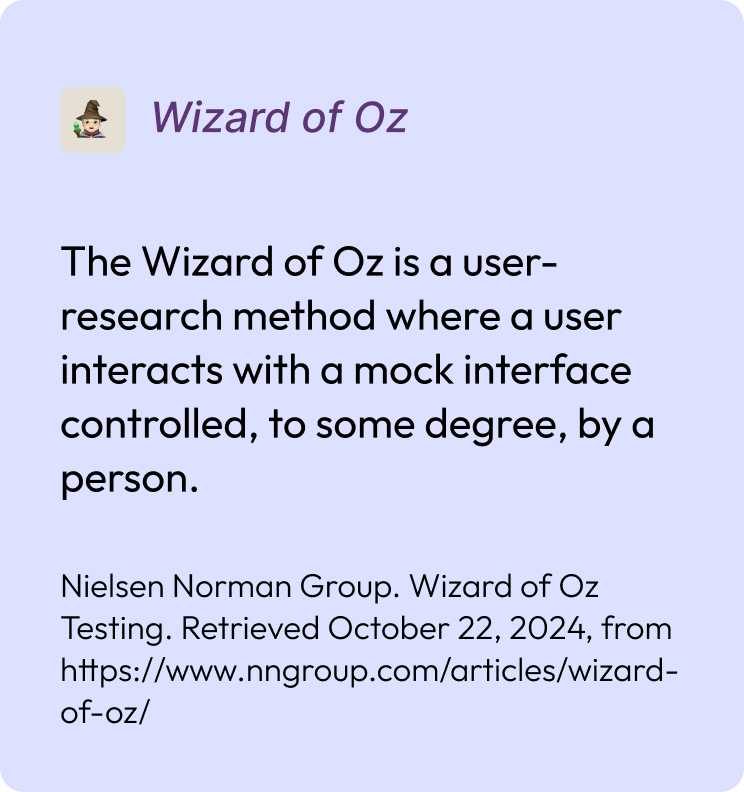

Testing these with students who regularly use Slack for coursework discussions allowed us to gauge whether these potential negative outcomes were significant enough to design for.
We started by analyzing all the feedback and grouping it into key themes with affinity mapping. From there, we focused on the fears that came up the most and felt the most significant, so we could design solutions that tackled what mattered most to our users.
01 FEAR OF TRUSTING AI
Participants were uncomfortable with AI analyzing their conversations, worried it might get things wrong, especially for international students.
02 NEED FOR VALIDATION
Users really wanted a way to check and confirm the AI’s information to make sure it’s reliable.
03 INACCURATE SUMMARIES
People were concerned that AI might misinterpret the tone of conversations, leading to wrong summaries and unfair flags.
04 LACK OF CONTEXT AWARENESS
Users doubted the AI’s ability to fully understand the context of their conversations and often needed to step in to clarify.
05 NEED FOR TRANSPARENCY
There was a strong desire for the AI’s decision-making process to be clear, especially when it comes to flagging sensitive content.
06 FEAR OF MISINTERPRETATION
The risk of AI misinterpreting and wrongly flagging conversations was a big worry, with potentially serious consequences for user engagement.
07 INVASIVE PROFILING OF STUDENTS
Users worried that AI might misinterpret private conversations, leading to unfair profiling or incorrect flags, which could seriously affect engagement.
PROTOTYPE • DESIGN ACTIONABLE CONCEPTS
Before we get to exploring our design concepts, let’s take a moment to understand some of Salesforce’s AI principles that guided our approach.
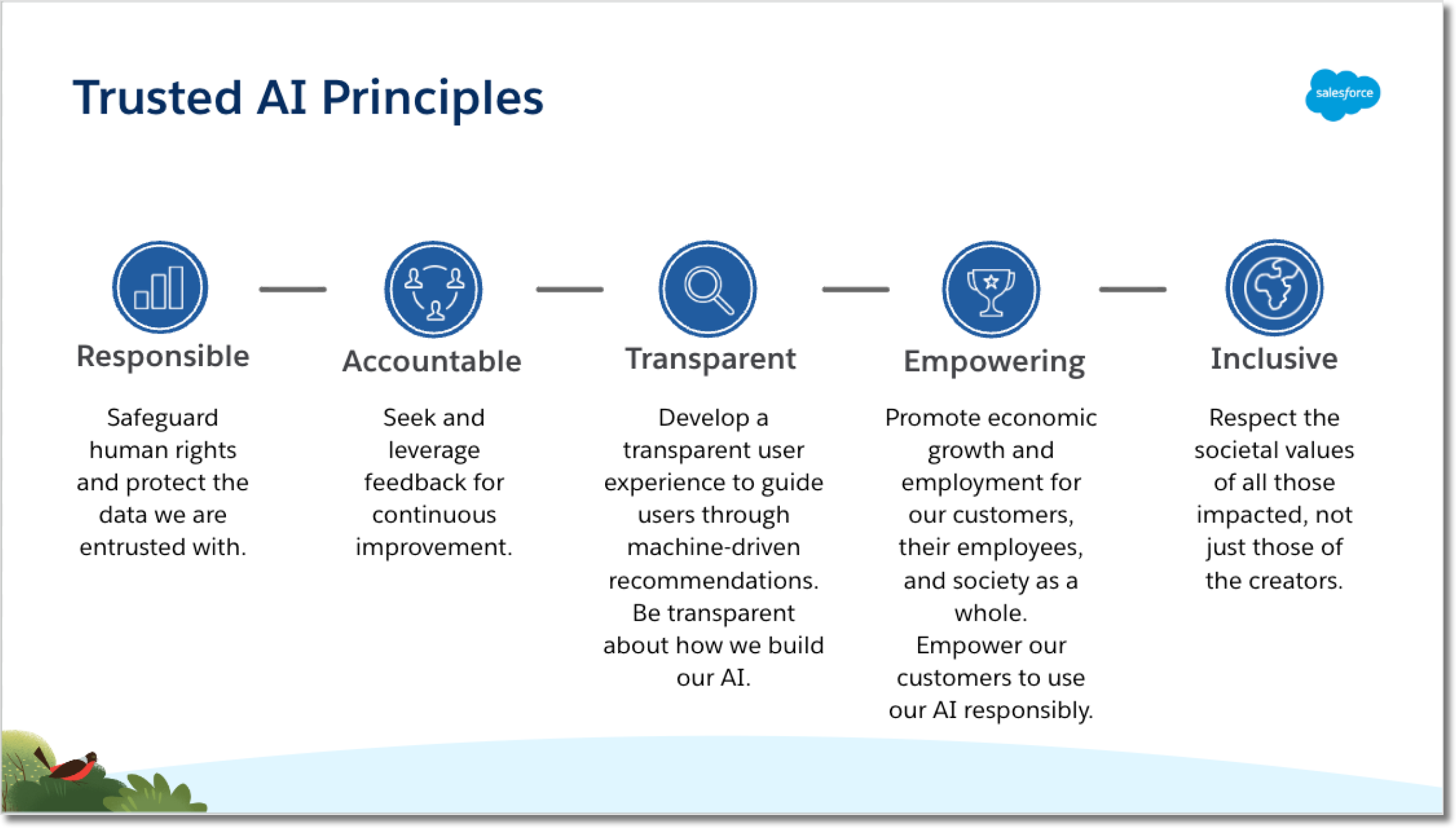
concept #1
in-line privacy alerts

When discussing personal challenges or sharing survey data,
students can "🔔 set reminders" to handle information carefully, ensuring confidentiality.
These alerts help students stay mindful of sensitive topics like personal data, supporting Salesforce’s Responsible AI principle by helping students protect their privacy.
custom alerts
Students can create custom alerts specific to their projects and discussions, setting up 📮user-initiated triggers for keywords that relate to sensitive topics.
Since they’re setting these alerts themselves, they’re more likely to interact with them and stay engaged.


concept #2
being transparent about AI’s bias moderation
This concept helps users understand that 🚩 AI can sometimes misinterpret context, cultural nuances, or language, leading to flagged content that wasn’t actually sensitive.
This concept aligns with Salesforce’s Transparent AI principle by giving users visibility into AI’s decision-making process and building trust through clarity and accountability.
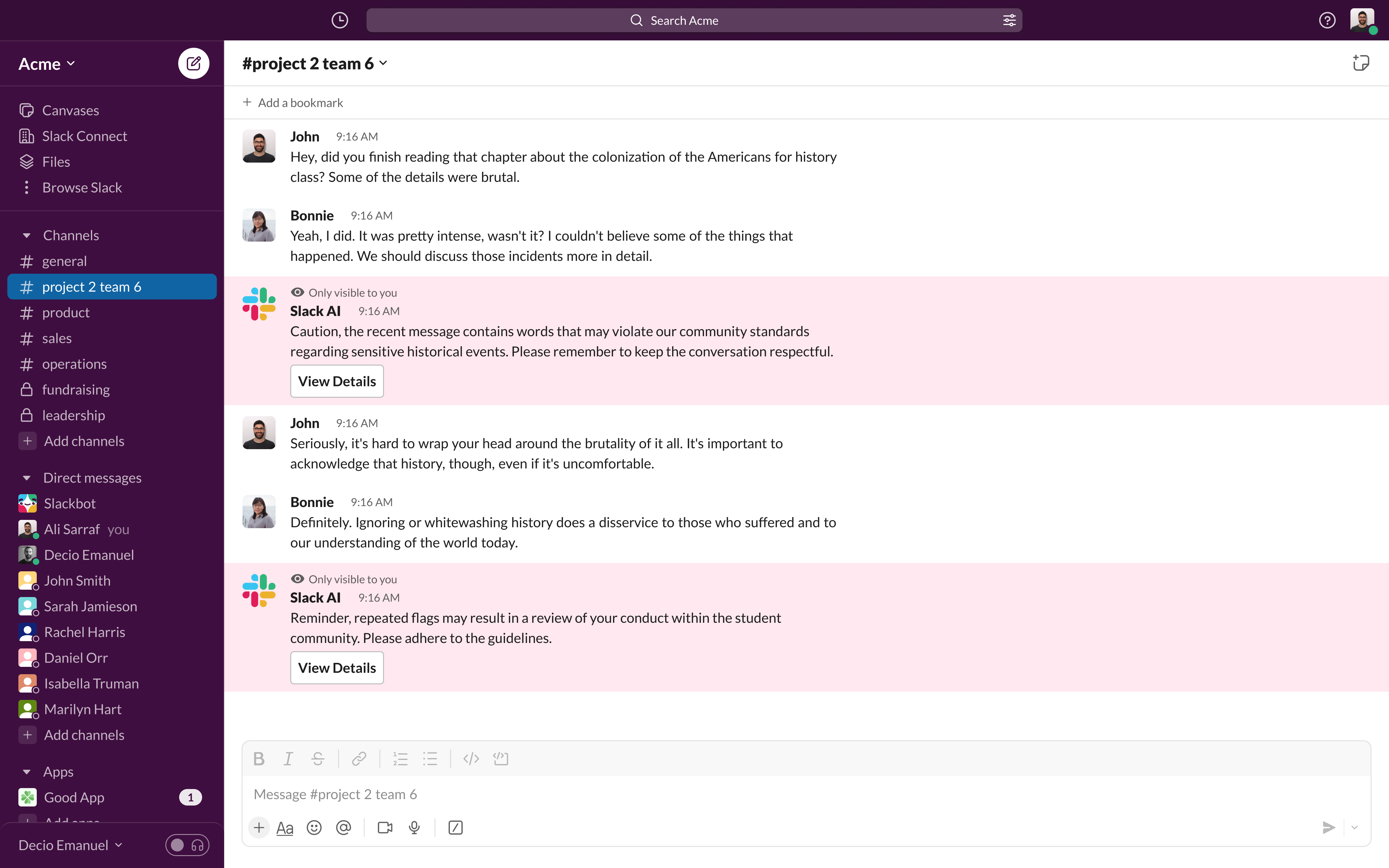
For example, if two students discuss America’s colonial history, the AI might flag it as “sensitive” without grasping the full context.
Users can easily see why their comment was flagged, helping them understand the AI’s decisions more transparently.
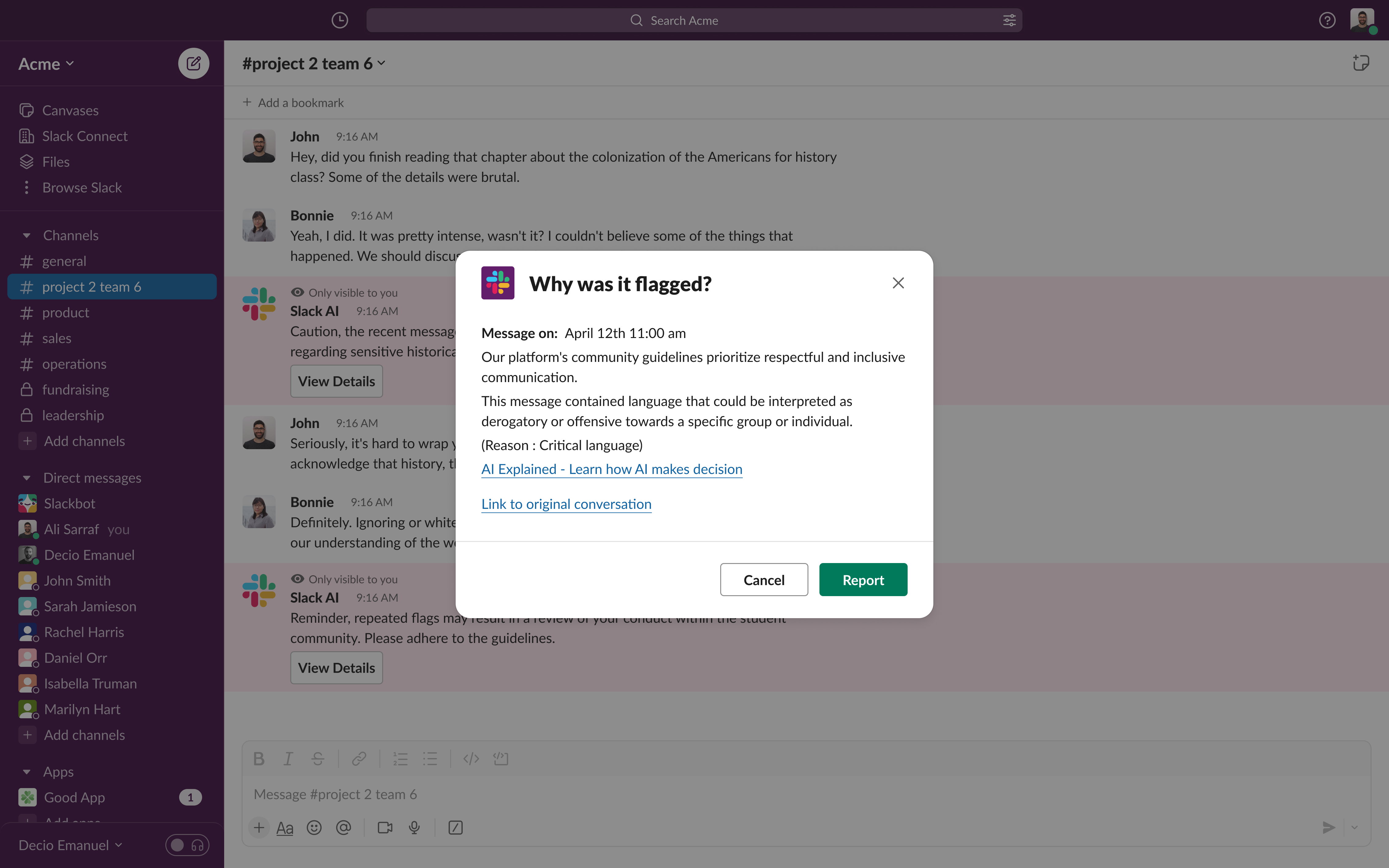
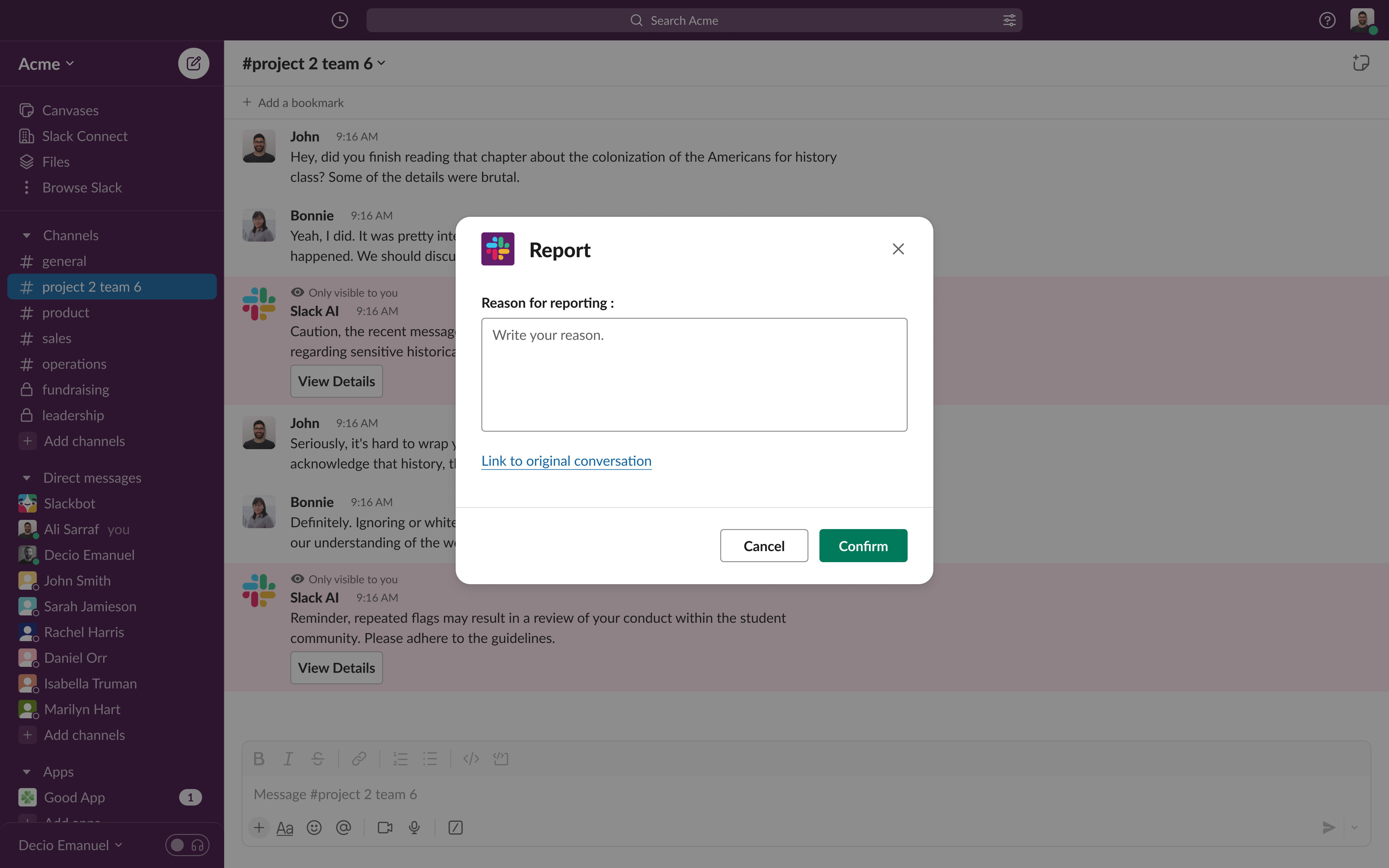
If they think it’s an error, they can review the flagged parts and explain their perspective. This option not only tackles potential AI biases but also helps build a fair, open environment where important topics are discussed freely in educational settings.
Allowing users to contest flags—whether they use it often or not—boosts their trust in the system, like 🛟 visible lifeboats showing that they’re in control.
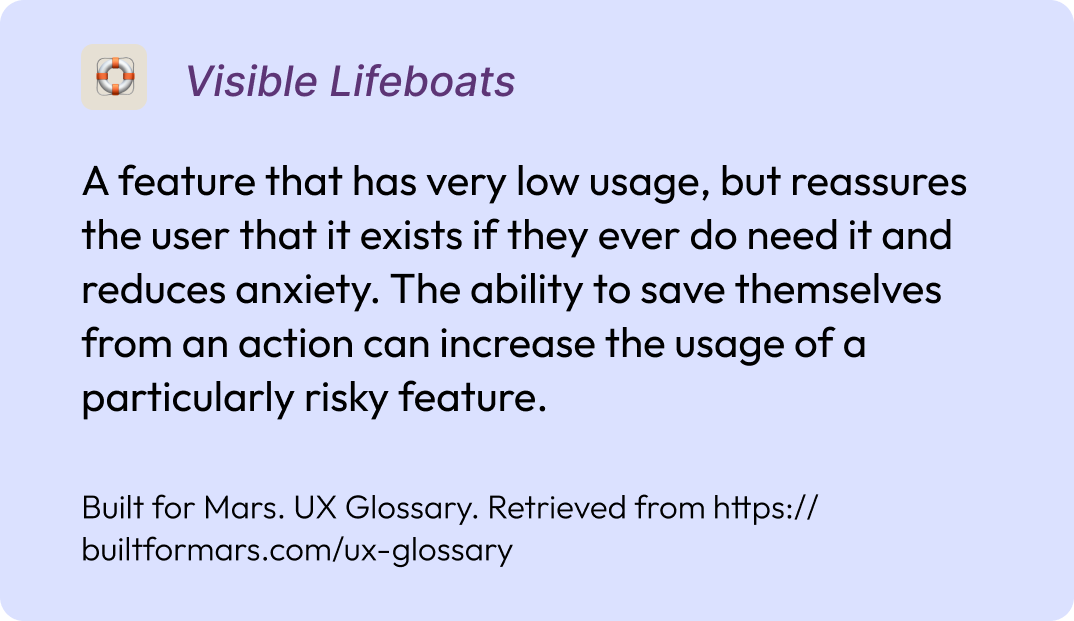
concept #3
misinterpretation ( incorrect summarization of conversations )
We recommend setting an “engagement style” for each channel. By selecting a style—such as formal, casual, or topic-focused— users enable the AI to interpret tone and intent more effectively, minimizing misunderstandings and enhancing the accuracy of summaries.
This feature
🫥 makes AI behavior more transparent by showing users how it processes discussions,
🫨 gives users control over the AI's tone and behavior, fostering a user-centered experience with context-aware, open interactions.
Here's a true story from one of our Slack channels. A student and professor were playfully teasing each other in the channel. This is how ChatGPT summarized the conversation:
🤖 “In this university class group chat, the professor expresses feeling hurt by De's actions and suggests points should be deducted. De responds dismissively, citing the professor’s age as a reason for forgetfulness.”
This shows how AI can completely miss playful tones, misinterpreting casual banter and taking things out of context.

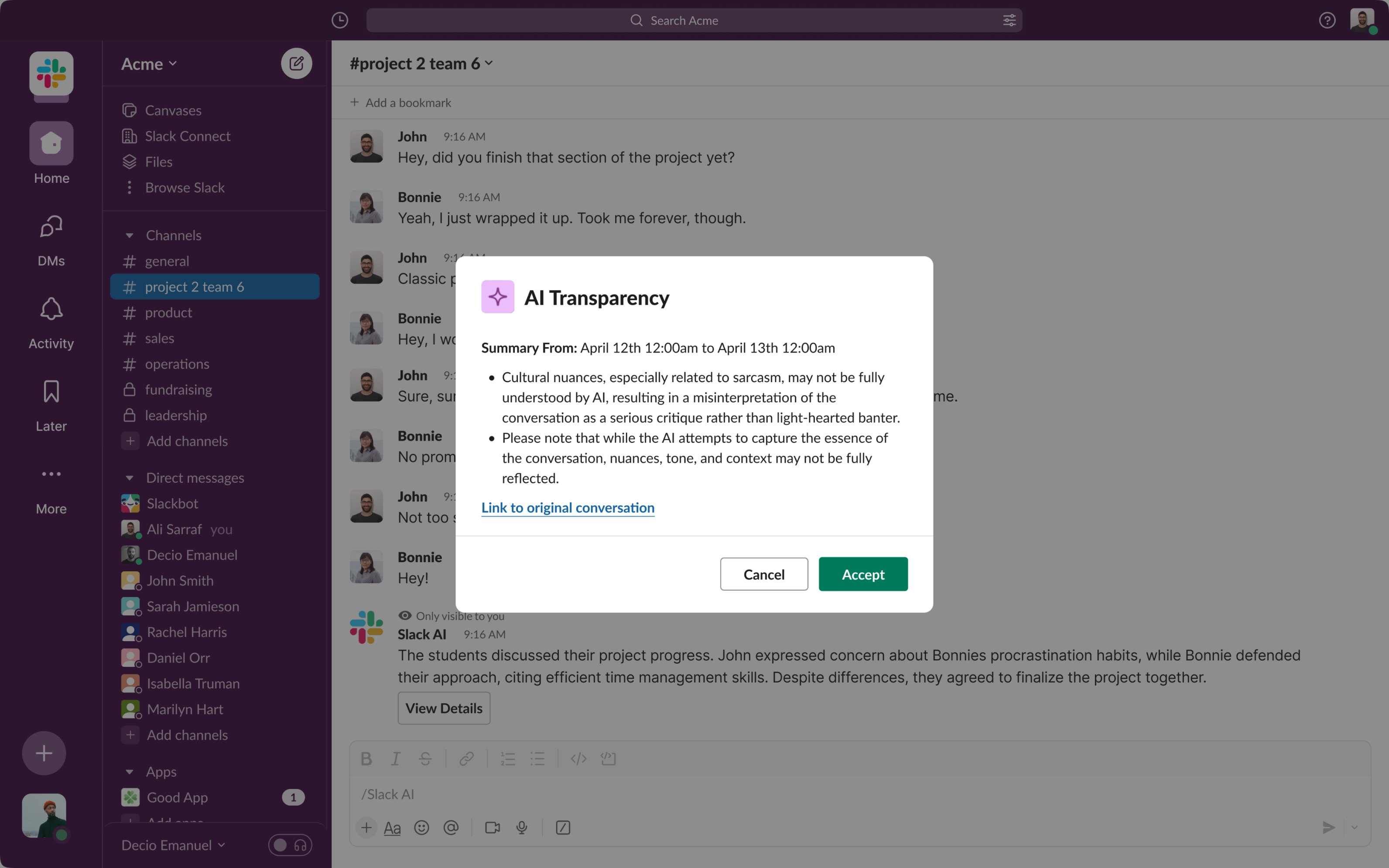
We suggested adding a user alert system to highlight AI’s limitations in understanding sarcasm or context when summarizing. This wasn’t a complete solution, but it helps set realistic expectations and minimizes misunderstandings when AI misinterprets casual or nuanced language.
Now, let’s see how our concept of setting a tone for the Slack channel helps.
Here’s a comparison of two summaries: one without setting a channel tone and one where an "engagement style" is defined as relaxed. You can see how adding this context changes the AI’s interpretation, aligning it better with the casual nature of the conversation.
By allowing users to set the engagement style, we give them more control over how AI captures the intent and tone of their discussions.
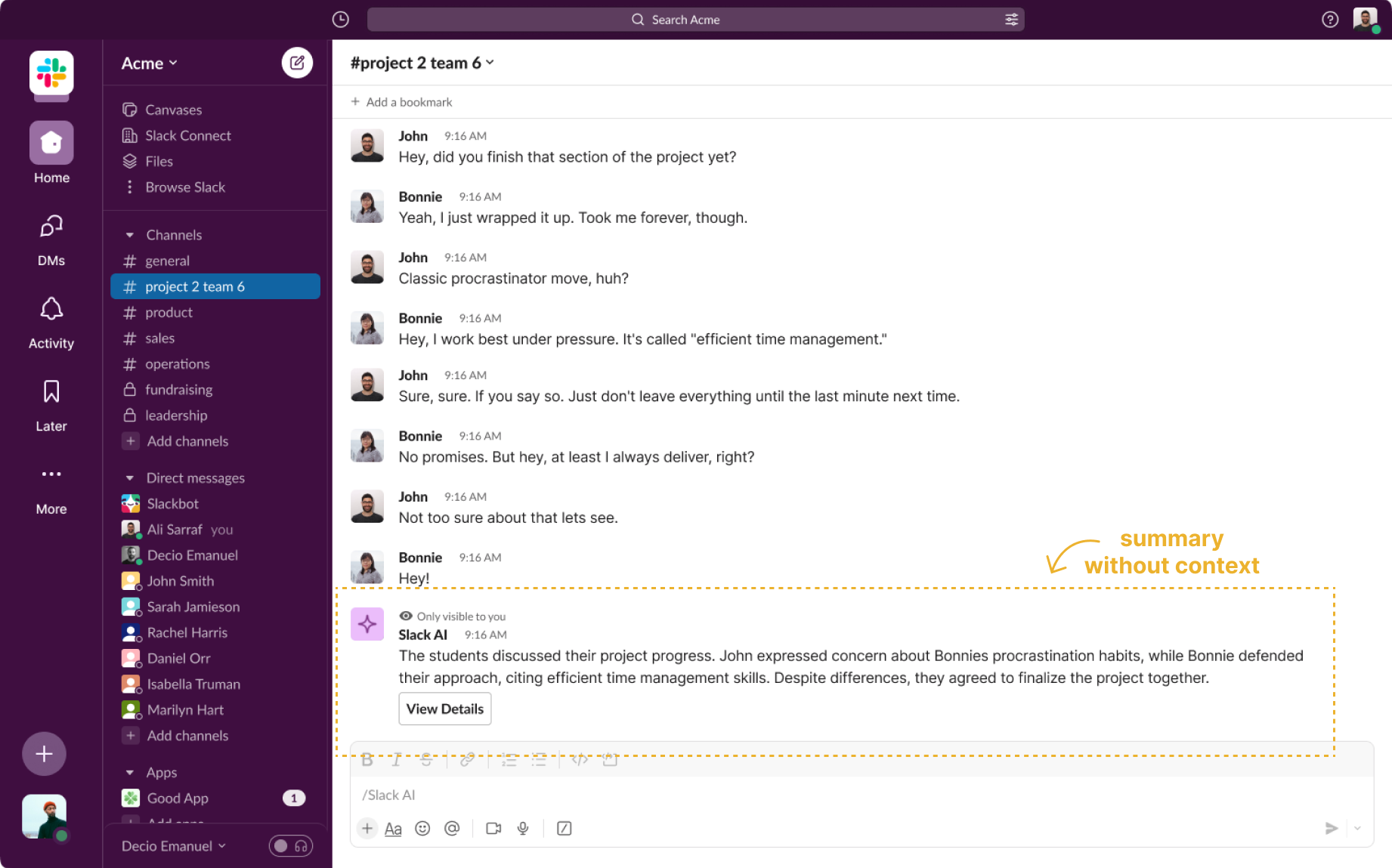
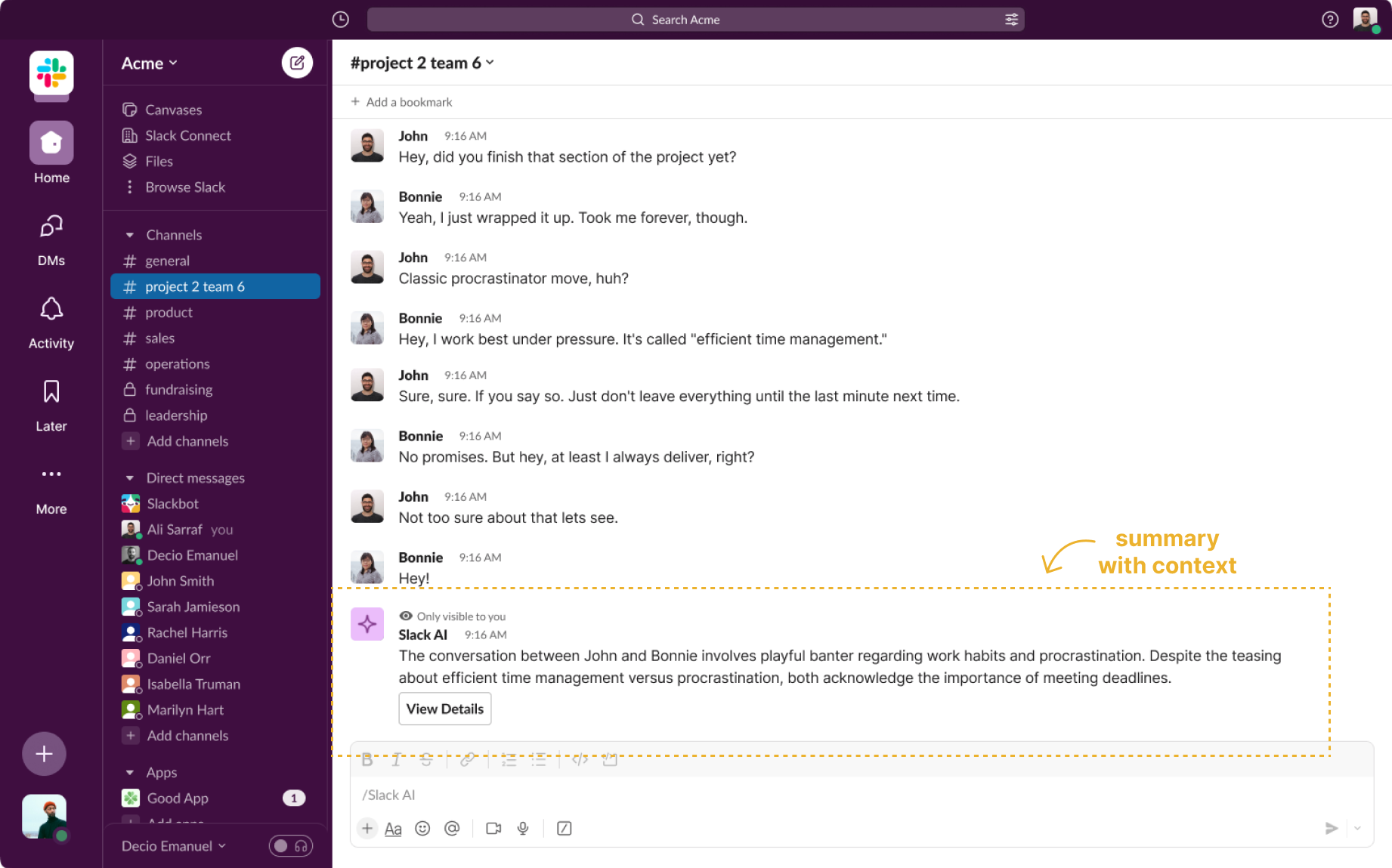
FUTURE WORK
01
02
We need to do extensive user testing for these design concepts. Currently, the channel tone settings may feel too rigid. For instance, if users realize that setting a “professional” tone could limit message flexibility, they might avoid it altogether. Plus, there are subtle nuances in group communication that the preset engagement styles may not fully capture.
Since this project centers on education, other roles—like instructors and researchers—need attention. For example, instructors monitoring student progress with AI could impact grades, so transparency with students is essential here.
OUR TRIP TO SALESFORCE
We were invited to the Salesforce office in Indy to talk about what we did, what our journey looked like, what hurdles we faced and how we overcame them. The people at Salesforce were really warm and invitiing and we had a great time!

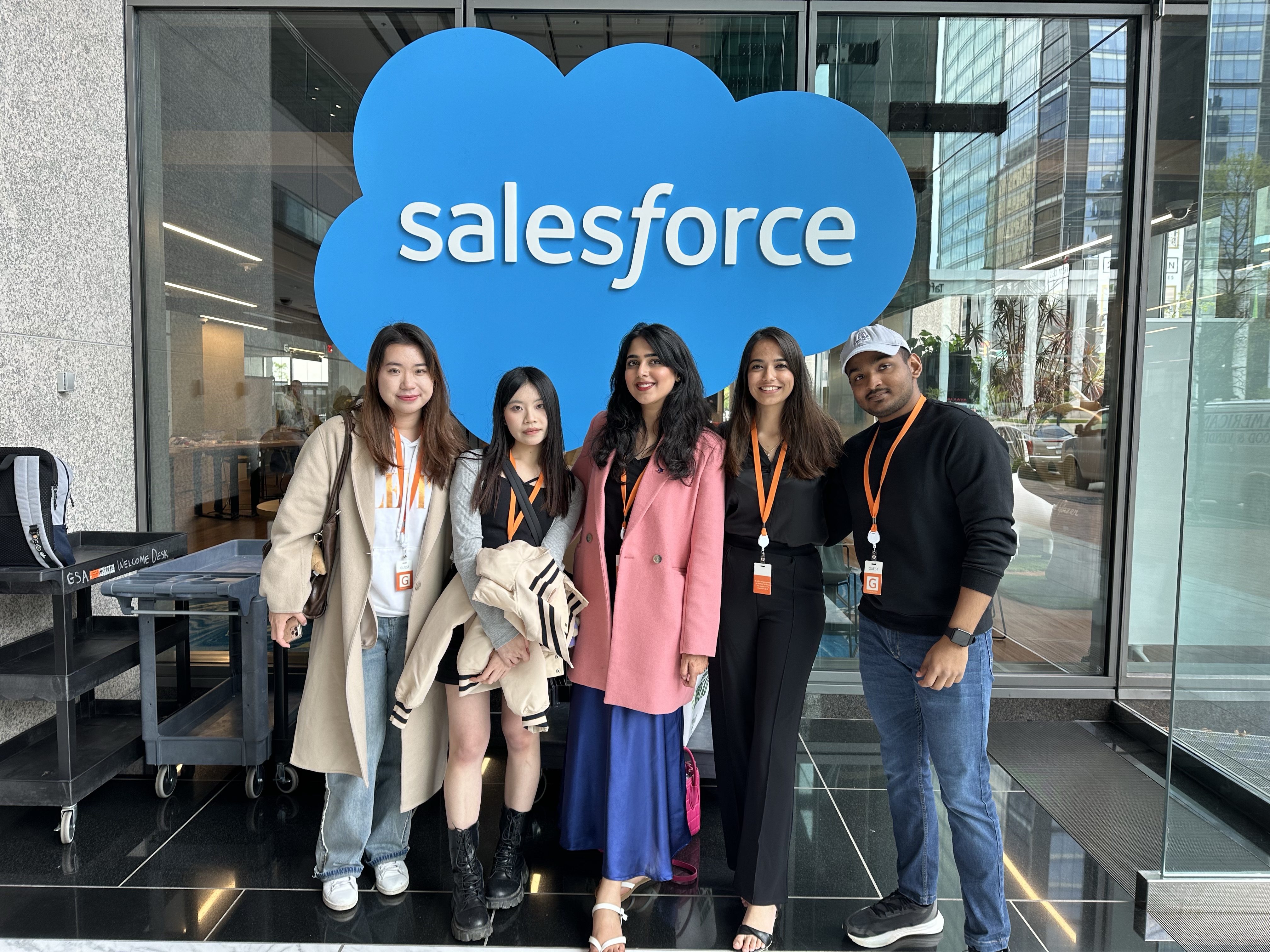
back to the top